
Image courtesy of Getty Images.
Latest News
October 13, 2023
Artificial intelligence (AI) is rapidly working its way into a number of simulation applications and workflows. Digital Engineering recently sat down with Ossi Saarela, Space Segment Manager at MathWorks, to discuss how AI can be used effectively in simulation workflows specific to space and aviation applications. (MathWorks offers a Deep Learning Toolbox for creating and training AI models for these types of scenarios.) Saarela is a former aerospace engineer at Boeing.
How does simulation influence and impact the design of AI models? What best practices does simulation bring to the development of AI models for space specifically?
Ossi Saarela: The space industry has a long history of simulation. I consider simulation a foundational technique for that industry, and one of the main reasons is that engineers can approximate but not fully mimic the space environment here on earth. The role of simulation is really important in the test and validation of space systems.
As AI models emerge and become incorporated more and more in the industry, they will also be incorporated into simulation. You have this convergence of AI as an emergent technology and simulation as a foundational technology, and I think in the space industry in particular, the AI models will, by necessity, have to be incorporated into simulation frameworks. So there will be a lot of influence that simulations play in the definition of AI models. The models will have to be built in such a way that they can be incorporated into simulation.
What are the common challenges that aerospace engineers face when implementing simulation into AI modeling? How can they overcome these challenges?
Saarela: There are a few different categories of challenges. One is that simulation and AI historically are two different domains of expertise, so folks that have experienced simulation tend to come with an aerospace background and tend to be well versed in controls and dynamics. AI comes from the software and data science domains. As AI is growing in the industry, we are finding that those two skills have to come together and cross pollinate.
MathWorks is seeing that aerospace engineers are doing a good job of picking up those AI domain skills.
Another category of challenge is when it comes to engineering tools. Tooling tends to be different for simulation and the AI, and some ways of bridging those gaps are using things like open standards for AI model sharing that allow those models to be brought into simulation environments. Mathworks has been investing heavily in enabling simulation of AI models in Matlab and Simulink either by building those models natively or importing them from other tools that data scientists have historically used.
In what ways does simulation help with integrating, implementing, and testing AI model components, as well as with reducing hardware costs?
Saarela: AI is software-native, so integrating the AI models into software-based simulation is much easier and quicker and more efficient than trying to integrate them into hardware-based test set ups. AI, being more of an emergent technology, has an inherent need for agile turnaround times for making changes to the models and re-running tests. Having an agile and faster-than-real-time test framework enabled by simulation has a lot of benefits over having a hardware-based test framework.
As engineers continue to find ways to develop more effective AI models for space, how do you see simulation and AI combining to solve common challenges?
Saarela: I think in the future it will be more and more common to have space systems simulations incorporate AI components. An AI component on its own does not provide the value that it does when it is incorporated into a simulation. There are a couple of different ways that AI can help solve challenges. One challenge it can help with is improving simulation performance; for example, computational fluid dynamics is a computational expensive process that is used in the space industry. And a one way to achieve better performance is by reducing computationally expensive physics-based models with an AI reduced order model that can approximate the CFD model at a required level of fidelity, but run much faster.
It can add capabilities to the system that are tough to establish or accomplish with traditional manually-generated algorithms. An example of that is using AI for vision-based sensing. In space you might use AI to perform visually complex tasks that require identifying scenes like docking on a space station or landing on a lunar surface.
What are some of the benefits of using simulation to augment existing training data?
Saarela: First of all, I love that you use the phrase “augment existing data” because one thing I think is critically important is to be able to anchor the simulated data to a ground truth so that you are training with data that has the right integrity, that has a basis in ground truth. That said, for some space applications you have to augment existing data with simulated data because there is simply not enough real data.
For example, the industry spent a lot of time around the Bennu asteroid and collected some images, but even those images need to be enhanced with additional simulated data for building AI models. Even in some cases where it is possible to collect data from a real system, it's often quicker and easier to use simulation to collect data.It is important to use models that are trusted, that have been verified and validated to generate that training data that can then be used to train AI models.
How do you see the future of AI models continuing to grow within the space industry? How can engineers best prepare to stay ahead of industry changes?
Saarela: It will continue to grow. Right now we are still in a very research-oriented phase for many of the AI applications. I think that as standards evolve to govern the verification/validation and use of these algorithms, we will see continued adoption.
Which aerospace applications would benefit most from simulation, and what examples can MathWorks share on the work it’s doing in this area?
Saarela: When we look at aerospace, the space segment is well positioned to benefit from simulation. Some examples of the work we are doing in that area are investing in making it easier to bridge the gap to bring AI models into simulation environments. We see the need there.
In a related vein, MathWorks is also seeing a lot of traction in the industry in multidomain, multiphysics simulation more generally where there is increasing interest in simulating interactions between different domains and subsystems – mechanical, electrical, thermal, and you can even throw in AI as being a different domain. These interactions can be pretty subtle and catch engineers off guard if those domains are simulated separately as opposed to together.
We have seen solutions that use AI to create reduced order models (ROMs) for early validation in the design process. Is that going to be a primary use of AI moving forward?
Saarela: That is a primary use case and even there, engineers should be a little bit careful in the early design phase. An AI model assumes that you have plentiful and accurate data about inputs and outputs of a system. For you to be able to build that model, you need a lot of data and understanding of the component you are modeling, so if you are doing early-phase design work you have to make sure you are not trying to model systems that are not very well understood using AI. instead you should bring in parts of the system that have some legacy to have enough data for training to be successful. For newer parts I encourage designers to use requirements-based behavioral models initially and then graduate to physics-based models, and as understanding increases, then that is the opportune time to build AI models.
What types of design and simulation data may be required for AI training, and will companies need to take new approaches to documenting and organizing their data to enable AI-based processes?
Saarela: One shift we are seeing in the AI evolution is that some of the emphasis is shifting from the design of the models themselves to the design and management of the training data. People are recognizing that the performance of the model is only as good as the data the models are trained on. Having the right quality and quantity of training data is critically important. Being able to solve that problem and manage that can be a real competitive advantage for companies. In my opinion, it is not just management of data sets, but there is also something to be said for having novel ways to create training data, especially from simulation in a way that it can be trusted. As standards evolve around AI in the industry, it's likely that a concept of some type quality metric or validation of training data will emerge.
More MathWorks Coverage
Subscribe to our FREE magazine,
FREE email newsletters or both!Latest News
About the Author
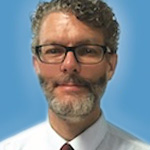
Brian Albright is the editorial director of Digital Engineering. Contact him at de-editors@digitaleng.news.
Follow DE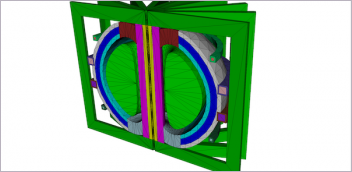
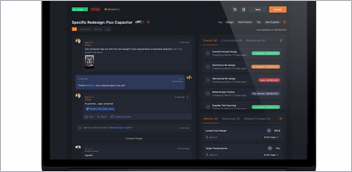
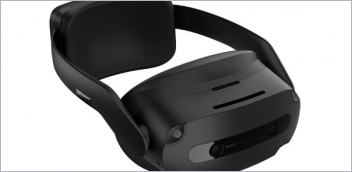
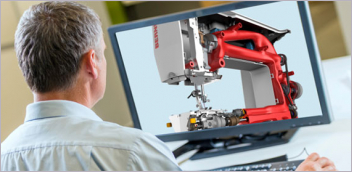